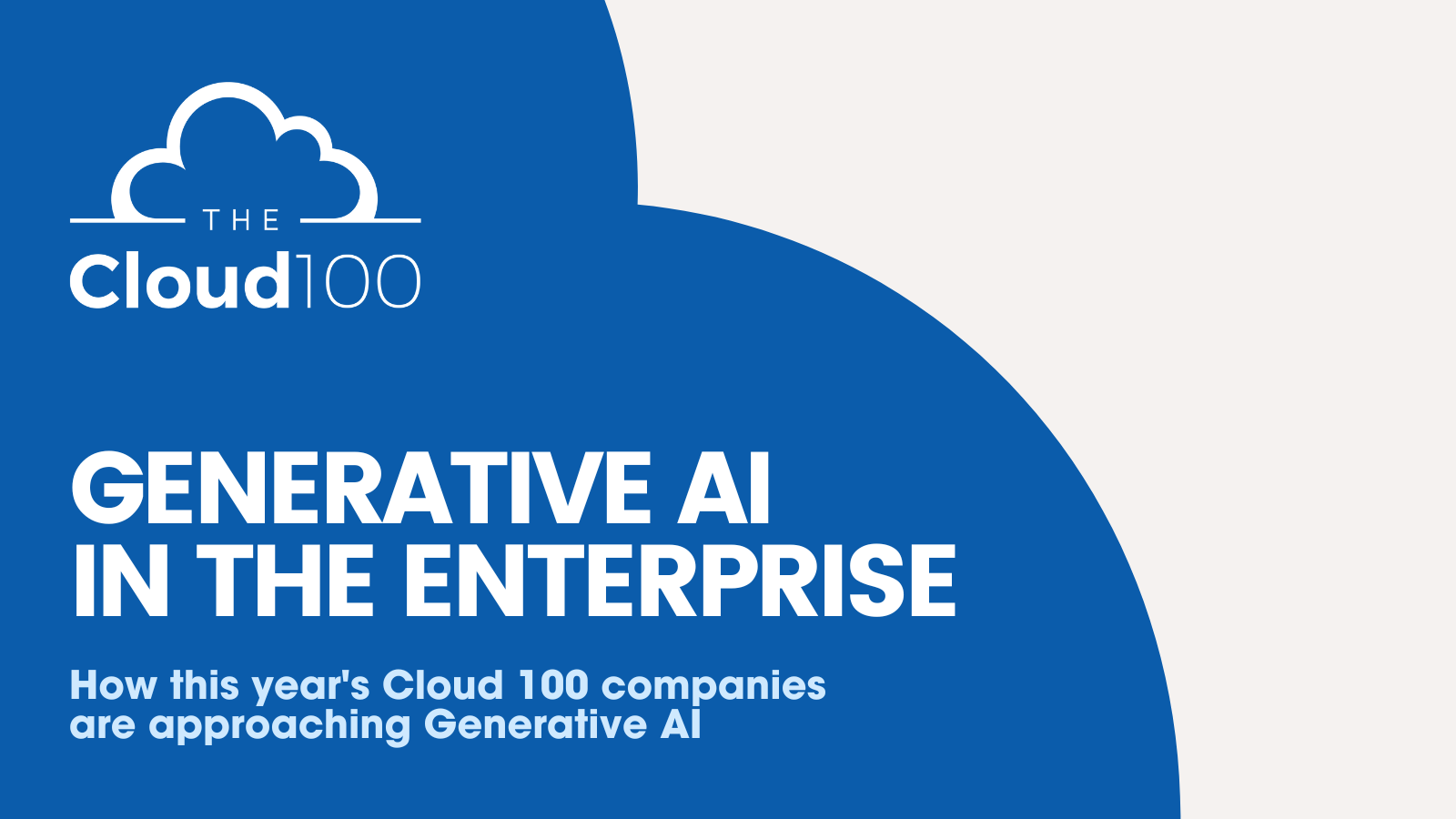
GENERATIVE AI IN THE ENTERPRISE
How this year’s Cloud 100 companies are approaching Generative AI
Globally, we are experiencing a profound technological shift — the rise of real-time data insights and generative artificial intelligence are shaping how we live, work, and interact. This is a seminal moment for AI.
The 2023 Cloud 100 list, produced in partnership with Forbes and Bessemer Venture Partners, recognizes standouts in tech’s hottest category from small startups to private-equity-backed giants, and this year it’s hard to ignore that AI is changing the way all companies think about their businesses.
The release of ChatGPT in the fall inspired the world to notice AI-powered functionality that had been building in the background among the research community for years. Almost immediately, consumers and knowledge workers began imagining a more creative and efficient future, and enterprises quickly felt the pressure to incorporate AI into their products and internal operations.
Then came the questions of where to start, how to build for the long run, and how to preserve trust and safety along the way. With a rapidly evolving market landscape, advancements in research and tooling launching nearly weekly, and differing opinions on the quality and performance of models, we’ve seen enterprises take a variety of approaches and this year’s Cloud 100 List is a perfect example of how cloud companies are approaching this new shift in AI:
BUILD VS. PARTNER VS. BUY: FOR PRODUCTION AND INTERNAL TOOLING
The rise of the cloud and modern data stack has provided enterprises with a valuable and accessible asset — their data. As it relates to Generative AI, enterprises have to balance leveraging this data to tailor models to their specific needs and use cases, while also protecting it. There are a few paths an enterprise can take. Let’s look at some pros and cons for each:
Use an existing frontier AI model such as OpenAI, Cohere or Anthropic
These models are often API-first and relatively easy to adopt, even for enterprises without dedicated machine learning functions. However, frontier models often lack visibility into underlying weights and pre-training data and require fine-tuning to serve more specific use cases.
- Notion, which is number 35 this year on the Cloud 100 lineup, has Notion AI, a product that is powered by OpenAI and Anthropic. By simply tapping the spacebar in any new line, users receive a prompt box that enables them to bring the power of the LLMs into an editable project page.
- Miro, which is number 10 this year on the Cloud 100, uses multiple models depending on the use case, including Microsoft Azure OpenAI Service and Miro’s internal models built on technology provided by third parties. Miro uses machine learning models along with user input to generate content on the Miro board.
- Gong, which is number 23 on this year’s Cloud 100 list, launched specialized Generative AI models in June that are powered by both frontier and internal models trained in-house using Gong’s unique customer-interaction dataset. The models are built specifically for revenue teams and are customizable.
Use an existing open model such as Meta’s Llama or Google’s BERT
Open models provide the benefit of being hosted locally on a company’s infrastructure. This approach requires more know-how of in-house infrastructure and ML expertise and still faces long-term memory limitations. However, the visibility into pre-training data and weights and the ability to train models for specific tasks are attractive enough for many enterprises to adopt.
The speed of innovation across the Generative AI landscape has been extraordinary in recent periods, with new open-source models launching weekly on Hugging Face, number 98 on Cloud 100 list. Moreover, Llama-2 was introduced last month on July 18th, which has the promise to change the open-source landscape for enterprises. By this time next year, we expect many of the Cloud 100 companies to have adopted open models as part of a multi-model approach.
Build a model internally such as Salesforce, Bloomberg, Databricks
Building a model internally allows companies full control over the pre-training data, parameters, and fine-tuning. However, this option can be expensive and challenging to maintain performance parity with publicly available models and requires in-house technical resources, expertise, and maintenance.
Cloud 100 veteran Databricks, which is number 2 on this year’s list, is betting that their data-science users prefer to build and finetune their own models, e.g., Dolly. Recently, they announced new Lakehouse AI innovations that allow customers to easily and efficiently develop Generative AI applications.
Leverage a product already fine-tuned for a specific use case
There is a cohort of emerging companies that leverage one of these model strategies, but go on to fine-tune for a specific use case, which can be attractive to businesses who may have less access to specialized training data or technical talent. Drawbacks of this approach may be implementation, limitations to company-specific customization, and potential loss of first-party data and feedback collection.
Cloud 100 Rising Star, Hearth, trains LLMs specifically on relationship workflows and network interactions to provide agentic relationship management. The result is an automatically updated and enriched view of one’s network that is proactive and actionable.
Cloud 100 Rising Star, Harvey, trains LLMs on legal information across every practice area, jurisdiction, and legal system to provide law firms and corporate legal teams with an AI assistant to tackle the most complex legal challenges like due diligence, legal research, and more.
BUILDING FOR THE LONG TERM: INFRASTRUCTURE, INTEROPERABILITY, CAPTURING FEEDBACK, AND FIRST-PARTY DATA
Many existing platforms have been quick to mobilize one of the aforementioned strategies in some form, and have done so at an impressive pace. The result has been some very neat features that have entered the market, most of which cover creative or predictable use cases that don’t require precision. As things stabilize, and we move into more nuanced, long-term products and tooling that depend on accuracy, how do enterprises build something sustainable? Here are a few key considerations:
- Collection of first-party data and user reinforcement: Even if enterprises aren’t hosting internal proprietary models, they need to be collecting and storing the feedback they’re receiving; so developing a long-term strategy is critical.
- Model interoperability: Many enterprises will leverage more than one model to serve a variety of use cases. However, there’s likely overlap both in the data the models are trained on and the respective feedback loops after they are deployed. Enterprises need to consider how to streamline infrastructure to control efficiency and cost.
- Fine-tuning vs. prompt optimization/engineering: Both are effective ways to get models to do what you want them to do. There is a tradeoff between performance and cost/technical expertise needed between the two options. Depending on the use case, one might be more suitable than the other. It’s important to build a strategy around which tasks require which method. The best approach could be combining both.
- Explore ways to ground models for better data retrieval: As companies are looking to solve the problem of hallucination and more accurate data in model outputs, one technique that has gotten more prevalent recently is Retrieval Augmented Generation (RAG). RAG is a technique in natural language processing where a generative model is combined with a retrieval mechanism to improve the accuracy and relevance of the generated text.
- Investing in hardware optimization: Compute continues to be expensive and its supply will remain limited in the near term. Investing in solutions that optimize for the utilization of hardware is important in this environment, similar to cloud optimization.
DEFINING AND MAINTAINING TRUST
AI gets a lot of things right but is still evolving technology and is not without risk. As businesses race to bring this technology to market, it is critical that they do so ethically and intentionally. It’s not enough to deliver the technological capabilities of Generative AI. Companies must prioritize responsible innovation to help guide how this transformative technology can and should be used.
Depending on an enterprise’s model strategy, they will have varying degrees of visibility and control into the underlying model but can apply additional controls to their own data & downstream, such as:
- Data privacy
- Traceability, attribution, and citation
- Guardrails on end-user prompting
- Monitoring for cyber attacks or misuse
This is undoubtedly a formative moment for enterprise software. Generative AI is revolutionizing the way consumers and enterprises interact with the world around them, and at an unprecedented clip. We are thrilled to see the Cloud 100 drive innovation in Generative AI across the model, infrastructure, and application landscape.
To explore this year’s Cloud 100 list, go here.