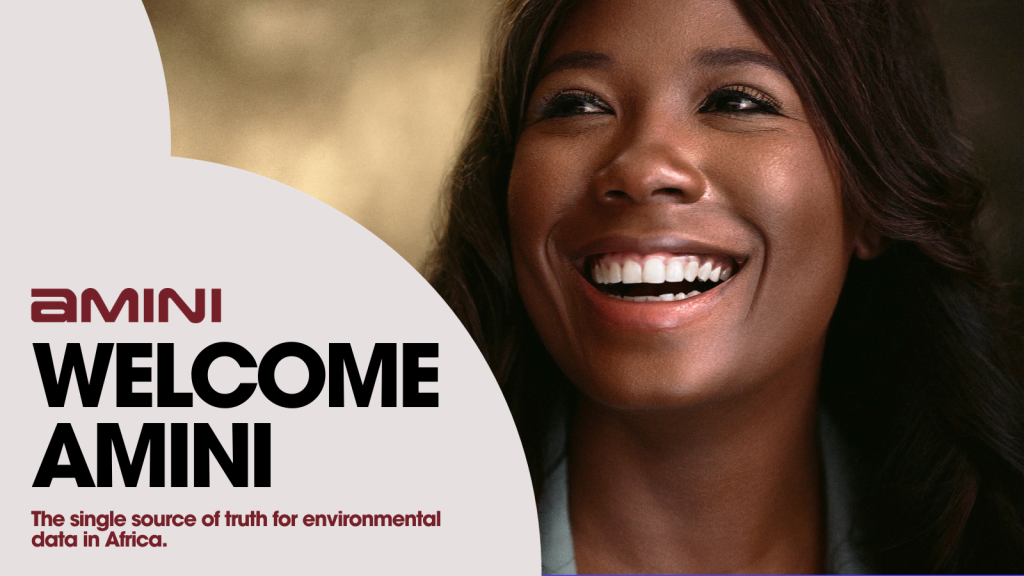
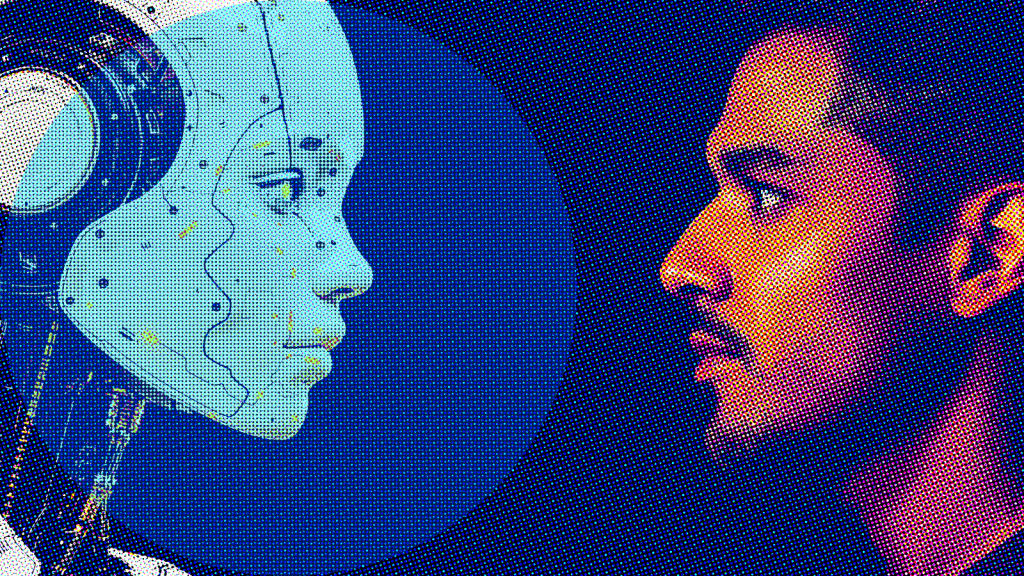
By the Numbers: How AI Will Impact Technology Work
A data-driven analysis of the impact AI will have on jobs in the technology sector.
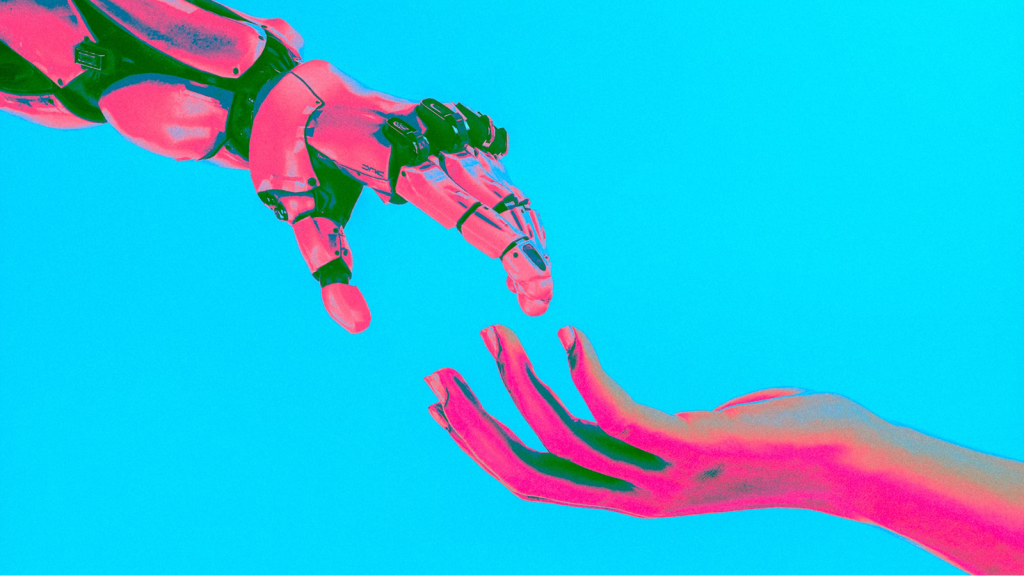
Building Trust in AI: 3 Approaches That Work
Lessons from Anthropic, GitLab, and Autogen AI.
Best practices for determining the impact of your AI efforts.
When Kate Jensen started in her role as Head of Global Revenue at Anthropic, she was tasked with measuring AI’s impact on her customers’ work — an increasingly common challenge across the industry given how AI can fundamentally change workflows.
“We were dealing with a technology that could potentially transform entire business processes, not just improve a single KPI,” Kate said at Dreamforce last year.
To measure AI impact, her team realized they had to go beyond simple ROI calculations and embrace the transformative nature of AI. In this blog post, we’ll dive deep on how to approach this essential challenge, offering lessons for any team or organization looking to determine the impact of their AI efforts.
It’s hard to know the “right” way to measure AI, and the technology is so new that waiting for guidance can mean missing crucial insights and opportunities. Anthropic’s team learned to start with imperfect metrics, refining their approach as they gathered more data and insights.
For example, when deploying Claude for customer service automation at companies like United Airlines and DoorDash, Anthropic initially focused on basic metrics like the number of customer service requests automated. This data provided a baseline and insight into where AI value is coming from.
Advice for founders: Begin with readily available metrics, but be prepared to evolve your measurement approach as you gather more data. Consider tracking:
Establish a 30-day baseline for these metrics before implementing AI. This gives you a clear “before and after” comparison to demonstrate impact.
It’s obviously important to look at the data, but Anthropic also benefited from customer feedback. Qualitative information helped Anthropic ideate benefits and use cases for Claude that weren’t evident from data alone.
Kate noted several concrete applications where Claude had delivered significant value for customers:
You can collect qualitative data through in-depth interviews with key users, stakeholders, and via focus groups. You can also analyze customer feedback and success stories, or use AI-powered sentiment analysis on user responses to identify potential trends or patterns that inspire product improvements.
As Claude adoption expanded, the Anthropic team realized they could go beyond those early, imperfect metrics. With a growing user base across numerous industries, the team had enough real-world usage data to identify meaningful patterns and outcomes. This critical mass of users helped the Anthropic team understand which metrics truly mattered for driving customer value.
The team worked to create a comprehensive set of KPIs — ones that captured the multi-faceted impact AI had across their user segments.
KPIs organizations may consider leveraging include:
You should create a scorecard for AI initiatives that captures impact across all these dimensions, and regularly adjust these metrics as AI capabilities evolve.
Tips for tracking KPIs effectively:
AI isn’t a “set it and forget it” tool. You need to constantly check on its performance, and make changes as needed. Claude’s success is a result of Anthropic’s commitment to continuous refinement based on real-world performance data.
We recommend the following best practices to quality-check your AI.
Also set up automated alerts for any significant deviations in key metrics, and schedule regular deep-dives to look for more subtle trends or emerging issues.
Initially, Kate shielded her team from less-flattering metrics to protect morale. But she realized this approach was hindering the team’s progress. By embracing full transparency, Anthropic opened the doors to faster problem-solving and more innovative thinking.
Ways to share AI performance data across your organization include:
As Anthropic’s journey demonstrates, when you measure AI’s impact, you should also consider continuous improvement, newfound opportunities, and staying ahead in a rapidly evolving field.
As you implement and scale AI in your organization, ask yourself:
With a comprehensive, nuanced approach to AI measurement — which embraces quantitative and qualitative insights, fosters transparency, and adapts with time — you can ensure your AI initiatives drive business value and give you a competitive advantage.
For a full guide to implementing AI effectively and efficiently in your organization, check out our new AI Implementation Playbook >>>